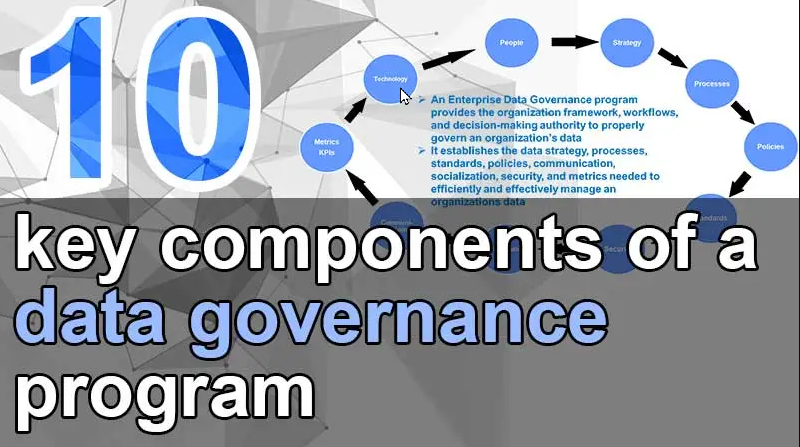
Data governance is the foundation of all data management programs. It is an essential disciple that supports all other data management knowledge areas like Data Literacy, Data Warehousing, Business Analytics, Big Data, Master Data Management, and many others. Data governance has 10 key components that exist to meet the enterprise’s data management requirements for each knowledge area.
People
Your data governance professionals, data stewards and other key business and IT staff are the backbone of your data governance program. They establish and develop workflows to ensure that your enterprise data governance requirements are being met.
Often organizations do not have the data governance skills that their program needs so they turn to a data governance consulting firm to assist in the mentoring and training with their data governance and stewardship staff. As a result, consultants and consulting firms are vital services for Data Governance programs. When working with a data governance consulting services firm its important to identify one who has a demonstrated track record of data governance success with clients who can attest to their governance services, solutions and capabilities.
Data Strategy
The data governance team plays a key role in the development and implementation roadmap of an organization’s enterprise data strategy. Building an enterprise data strategy is a vital step in the data management journey; however, many organizations fail to understand the need for a formal and documented data strategy. A data strategy is an executive document which provides the high-level, enterprise requirements for data and a strategy to ensure that those requirements will be met.
Data Processes
Data governance programs need to establish key data processes for data management. Common policies include data issue tracking/resolution, data quality monitoring, data sharing, data lineage tracking, impact analysis, automated data quality testing, and many others.
Data Policies
A data policy is a high-level set of one or more statements that will state expectation and expected outcomes, to influence or direct data habits at an enterprise level. Data Governance programs establish data governance policies for data management. Policies include outbound data sharing, regulatory adherence, and many others.
Data Standards & Data Rules
A data standard provides a framework and overarching approach for ensuring adherence to a data policy. An example of a data standard could be the use of the ISO 3166 standard for the definition of the codes for the names of countries, dependent territories, special areas of geographical interest, and their principal subdivisions.
A data rule directs or constrains behavior to ensure adherence to data standards which, in turn, provide compliance with data policies. An example of a data rule would be an organization having a rule that only country codes that are listed in the ISO 3166 Standard. Typically, organizations will look to establish data rules for master & reference data, data definitions & domain development, metadata management, classification, accessibility, and many others.
There are enumerable data standards that a data governance program can leverage. Some of the more notable data standards of interest are:
- ISO (International Organization for Standardization): Many are available!
- 3166: defines codes for the names of countries, dependent territories, special areas of geographical interest, and their principal subdivisions
- 19115: An internationally adopted schema for describing geographic information and services. It provides information about the identification, the extent, the quality, the spatial and temporal schema, spatial reference, and distribution of digital geographic data
- Dublin Core: A basic, domain-agnostic standard which can be easily understood and implemented, and as such is one of the best known and most widely used metadata standards
- Human Resources (https://dw.opm.gov/datastandards/list)
- Geospatial (https://www.fgdc.gov/standards/standards_publications)
- Genome: consists of 61 different metadata fields, called attributes, which are organized into the following seven broad categories: Organism Info, Isolate Info, Host Info, Sequence Info, Phenotype Info, Project Info, and Others (http://www.dcc.ac.uk/resources/metadata-standards/genome-metadata)
- Protocol Data Element Definitions: Used in Clinical Trials
- Health Level Seven: provides standards for exchanging clinical data.
- Continuity of Care Record: responds to the need to organize and make transportable a set of basic information about a patient’s health care that is accessible to clinicians and patients.
- SNOMED: Systematized Nomenclature of Medicine Clinical Terms – provides comprehensive computerized clinical terminology covering clinical data for diseases, clinical findings, and procedures.
- Need a giant list of standards? (http://www.dcc.ac.uk/resources/metadata-standards/list)
- Do you just want healthcare standards? http://healthstandards.com/blog/2006/10/11/what-are-the-different-standards-in-healthcare/
Data Security
Data security involves protecting digital data, such as those in a database, from destructive forces and from the unwanted actions of authorized and unauthorized users, such as espionage, cyberattack or a data breach. Most Global 2000 companies have entire teams dedicated to data security.
Communications
Data governance communications include all written, spoken, and electronic interaction with association audiences who have interest or need to know about the activities of the data governance team. A communication plan encompasses objectives, goals, and tools for all communications, and should be part of a governance program from the early part of the program’s development.
A communications plan is the key artifact of enterprise data governance communications. The plan identifies how to present governance and stewardship challenges and successes to the various stakeholders and to the rest of the organization. The communications plan highlights the right business cases and presents their results.
A successful communication plan is built on strong themes and is more effective than one with unrelated and scattered messages. The plan encompasses objectives, goals, and tools for all communications that the Data Governance program requires.
Socialization
Socializing a program such as governance is essential since it is a departure from the status quo. This new approach to properly governing the enterprise’s data must be incorporated into each area of the organization and made part of the “culture”. Socialization of data governance is a continuing process, and it is an important activity in any governance program.
The data governance socialization plan is the cornerstone of data governance socialization. The data governance socialization plan is a plan and process by which data governance activities and integrated into an organization's policies, internal culture, hierarchy, and processes. The plan is unique to the organization as it must be tailored to the enterprise’s culture and standards of behavior.
Education and certification are vital vehicles that an organization can use to socialize data governance and data stewardship. The most optimal data governance training is through online and on-demand courses which have the capability to measure the progress of each data governance professional. These certified data governance education training courses should be mobile-enabled and available 24/7 so that the staff member doesn’t have to leave work for weeks on end to earn their certification. Organizations need to heavily invest in training and education to ensure that the people component of data governance provides the maximum amount of value to the enterprise.
Metrics/KPIs (Key Performance Indicators)
Establishing business metrics and KPIs for monitoring and measuring overall business impact of the data governance program is vital to the program’s success. The metrics and KPIs need to be measurable, tracked over time, and consistently measured the same way year in and year out.
Many organizations make the mistake of not taking a baseline measurement and then, after years, trying to go back and measure their data governance program’s success. These situations make it very difficult to come up with an accurate measurement of success.
Technology
The data governance program will need various technology in order to be as automated as possible. Smaller data governance programs will typically use the technology stack which they already have within their enterprise. Larger data governance will purchase software that is specific to data governance and the functions it requires. Data governance software simplifies the process of scanning databases & files to capture needed metadata, management of the metadata, automating the data stewardship workflows, decision trees, social voting, collaboration, and many other data governance and stewardship functions.
For an organization to be successful at enterprise data governance it needs to actively build the 10 key data governance components discussed in this article. Each component requires skilled data governance professionals and seasoned data stewards to fully implement.
--------------------------------------------------------------
Credit by 10 key components of a data governance program | LightsOnData